It is hard to imagine the field of modern medicine without the development and harnessing of technology. X-rays, MRIs and other solutions have been widely utilized in various medical fields to improve diagnosis accuracy and efficiency, whilst also minimizing instances of human error.
As technologies continue to develop, novel implementations of various technologies and breakthroughs are both anticipated and indispensable. One example of such technologies is AI image recognition models that can be trained to offer versatile and significant uses within medicine.
This article provides examples of how image recognition models can be used to improve the accuracy of medical diagnoses, to assist in retrieving information on similar conditions, and to even prompt people to seek medical advice based on a preliminary AI-powered diagnosis.
What Is AI Image Recognition?
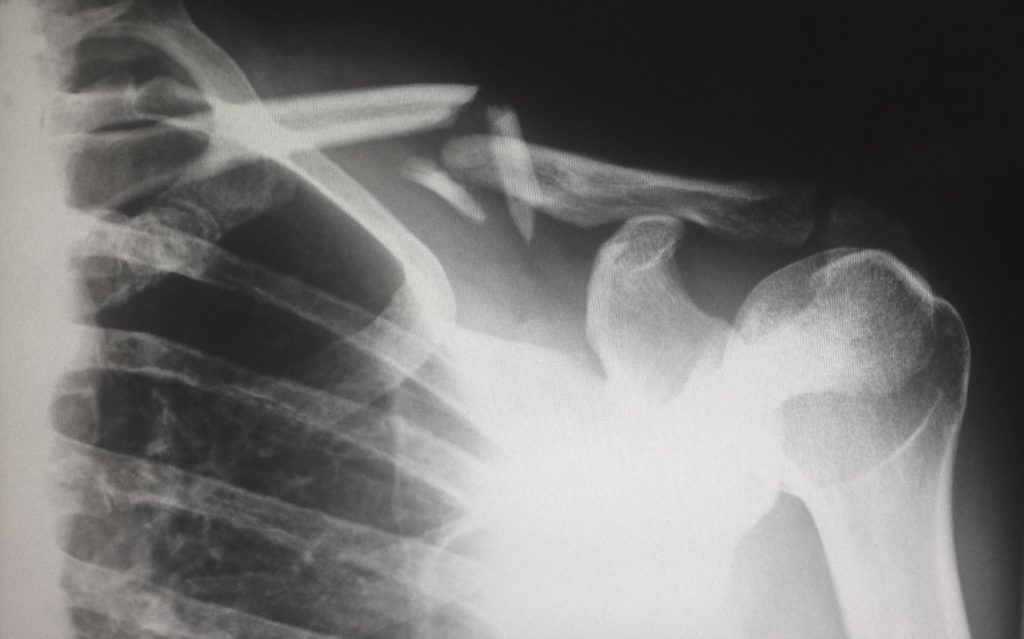
AI image recognition is a subfield of deep learning that uses computer vision to identify the content of images. The three primary image recognition model types include:
- Image classification
- Object detection
- Image segmentation
Whilst all three deploy similar neural networks to identify the content of images, they all serve different purposes. Within the field of medicine and healthcare, image recognition models can be trained to spot, identify and/or locate specific instances within images, such as defects, tumours or lesions.
How can AI image recognition be used in medicine?
Diagnostics
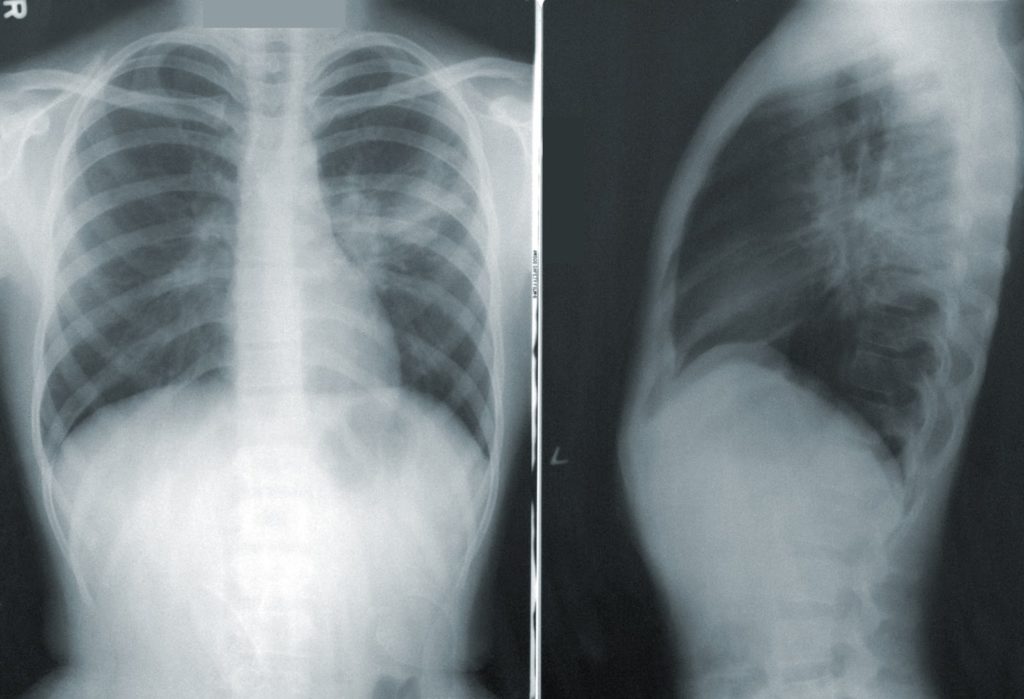
Image recognition models can assist in the diagnosing of various conditions. The models can be trained and deployed to scan images from MRI or X-ray machines, as well as other visual outputs, to detect, locate and flag up medical abnormalities that the model has been trained to identify.
For example, it can identify the number and exact locations of tumours within an image, helping to direct the attention of the medical practitioner to the malignant or cancerous elements.
Such information can help doctors in providing timely and accurate diagnosis which may assist in the patients’ course of treatment. It can also increase their ability to identify small malignant elements (e.g. tumours) which may not be clearly visible to the human eye. This prompt and accurate diagnosis would in turn improve the efficiency of medical services and providers, decrease the time dedicated to screening and rescreening, and add to doctors’ ability to provide an early and accurate treatment plan.
Such benefits would be crucial especially for doctors dealing with large-scale and unusual events that often-put high pressure on emergency rooms (e.g. victims of natural disasters, wars, etc.). Additionally, the image recognition models can be trained to one centralized standard, and deployed across a wide range of hospitals and clinics, helping to standardize the diagnosis process across regions.
Training and human-error prevention
Trained image recognition models can also assist doctors’ training. The models can be a supporting mechanism for junior doctors to ensure that they have not missed important details within X-rays or MRIs during diagnosis and interpretation of screenings. Platforms such as SentiSight.ai offer project management functionalities that allow supervisors to easily review the deployment of the image recognition models, creating a safety net for junior doctors to use the models, with the more senior management able to ensure everything operates as it should. Not only does this raise the standard and quality of the diagnosis processes, but it also helps to reduce the stress young doctors might feel as they work to diagnose patients correctly.
This is equally applicable for dentistry fields that use X-ray scans to identify whether individual teeth need invasive treatment. In certain instances, various conditions can be hardly noticeable, especially at the early stages of their development (such as decay). Object detection models can be trained to scan images taken by X-ray machines and direct dentists’ attention to potentially problematic areas.
Moreover, these AI-powered models can reduce the amount of lawsuits, financial repercussions, and reputation concerns that hospitals encounter due to human errors. According to Pinnacle Care, human errors and other inefficiencies in the US health sector cost $750 billion each year and most Americans get misdiagnosed at least once in their life. The annual deaths related to such errors in the US are between 40,000 and 80,000 which indicates the need for advanced technological solutions that could help to counter this problem. Technology such as image recognition models within medicine can help to standardize processes, as well as creating an accountable trail of processes and diagnoses.
Retrieving information on similar conditions
Another way to utilize image recognition models is through using this AI-driven technology to help retrieve information on similar conditions that have been identified to be closely related in any number of ways to the patient’s results from a medical examination. MRI or X-ray scans are already implemented to provide medical teams with insightful images for a wide array of various diseases and traumas, including cancer, broken bones, and many other conditions.
Image similarity search can be beneficial in this field by retrieving similar images to the ones being analyzed, taken from the patient’s medical examination. This can help assist doctors with an accurate and correct diagnosis by image similarity search models supplying X-ray/MRI images of a similar physical appearance to those images from the patient of concern.
Such insights would be highly beneficial for a couple reasons. First, they would reduce the probability of human error in case doctors miss some important details present in images. Second, insightful information could be appreciated by less experienced doctors at the early stages of their careers.
Early diagnosis
SentiSight.ai software can also be employed for medical purposes outside hospitals. AI image recognition models can be trained by medical professionals to the highest standard, and then deployed by layman operators with basic imagery capabilities.
For example, image recognition models can analyse images containing birth marks or any other skin pigmentation changes and suggest the probability of skin cancer, infections, or other conditions. Based on the resulting analysis, the models can suggest whether the person should seek medical help or not, based on the probability thresholds dictated by the trained medical professionals who helped to develop the models.
The implementation of such technologies as a self-diagnosis medium beyond hospitals could increase the number of people who would regularly keep track of their health condition and flag any potential issues at a very early stage.
SentiSight.ai’s image recognition platform consists of three models, ideal for use within the medical and healthcare field:
- Image Classification Models – classifies the content of the image into specific categories and provides a % confidence estimate of each classification label.
- Object Detection – scan, identify and locate the content of images.
- Image Similarity Models – deployed to find visually similar images within large datasets.
Conclusion
Overall, image recognition models such as those built and deployed upon SentiSight.ai can be highly beneficial in the medical sector. When used to improve the accuracy and speed of medical diagnoses, the image recognition models bring significant health benefits to patients who benefit from early treatment. Additionally, they also deliver significant financial benefits for hospitals by reducing the probability of human error and the expenses incurred by misdiagnosis.
They also support medicine students or less experienced doctors during their training or when embarking on their careers. Outside the hospitals, once trained by medical professionals these models can be used to identify the probability of various conditions and prompt people to seek medical attention in the case of a potential abnormality. If you have an idea of how to incorporate image recognition into your healthcare operation, SentiSight.ai is your place to turn an idea into reality. Our online platform consists of powerful yet user-friendly AI-assisted tools to help anyone, whether you are a beginner or an expert, to build, train and use your own image recognition models.
Check out how image recognition can be utilized in other industries like retail.